Unlocking the Power of Data Annotation: A Comprehensive Overview
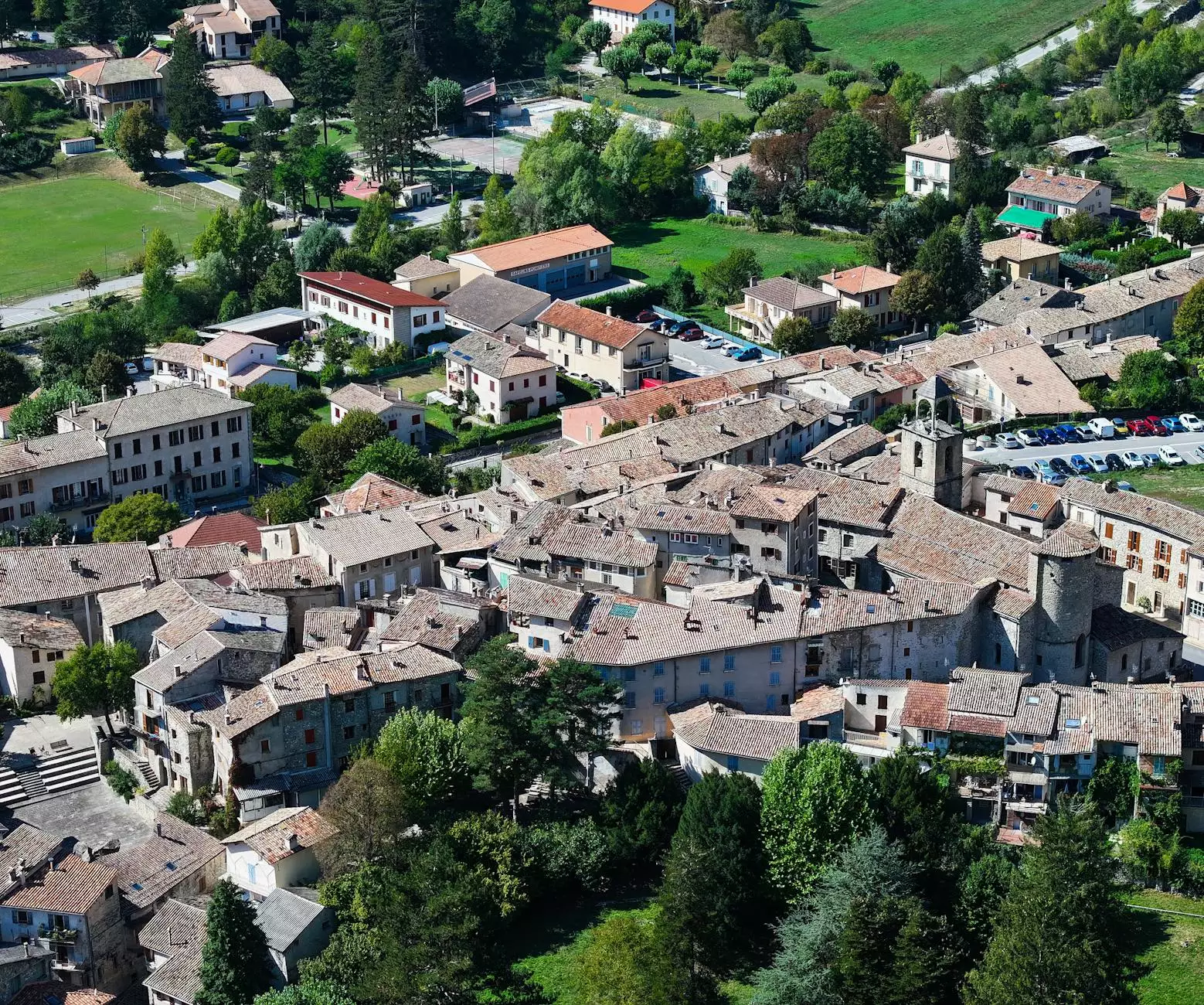
Data annotation is an essential process in the realm of artificial intelligence (AI) and machine learning (ML). It involves labeling and tagging data to enhance its value and usability for training AI models. As companies across various industries strive to leverage AI technologies, the demand for efficient and high-quality data annotation tools and data annotation platforms is skyrocketing. In this extensive guide, we'll explore why data annotation is vital, the different types of tools and platforms available, and how Keylabs.ai stands out in this competitive market.
Understanding Data Annotation
Data annotation can be described as the practice of adding informative labels to data sets. This process is crucial for machine learning models, as annotated data serves as the backbone for training algorithms to recognize patterns and make informed predictions. Without adequate and precise annotations, the effectiveness of AI applications can be severely hindered. Some common types of data that are annotated include:
- Text Data: Involves labeling parts of speech, sentiment analysis, and entity recognition.
- Image Data: Pertains to identifying objects, image segmentation, and facial recognition.
- Audio Data: Consists of transcribing spoken words and identifying different sounds.
- Video Data: Involves tagging movements, actions, and specific objects within a video stream.
The Importance of Data Annotation in AI and ML
As AI technologies continue to evolve, the significance of high-quality data annotation cannot be overstated. Here's why effective data annotation matters:
- Improved Model Accuracy: High-quality annotated data enhances the learning process of machine learning models, leading to improved predictions and outcomes.
- Faster Deployment: Efficient data annotation processes expedite the development cycle, enabling companies to launch their AI solutions faster.
- Cost Efficiency: Automated and well-structured data annotation can significantly reduce costs associated with manual labeling efforts.
- Scalability: A robust data annotation platform allows businesses to handle larger data sets as their requirements grow.
Key Features of a Reliable Data Annotation Tool
When evaluating data annotation tools and platforms, several key features should be considered to ensure they meet your organization's needs:
1. User-Friendly Interface
A well-designed interface allows users to navigate the tool with ease, ensuring that both technical and non-technical staff can utilize it effectively.
2. Versatile Annotation Capabilities
Look for tools that can handle various data types, including text, images, audio, and video, providing flexibility for diverse projects.
3. Quality Assurance Mechanisms
Incorporating quality control measures, such as review processes and feedback loops, ensures that the annotations are accurate and reliable.
4. Collaboration Features
Multi-user functionality that facilitates teamwork is crucial for larger projects that require input from different stakeholders.
5. Integration Capabilities
The ability to integrate with existing systems and data pipelines enhances the usability of annotation tools within your current workflow.
Exploring Keylabs.ai: A Premium Data Annotation Platform
In the vast landscape of data annotation tools, Keylabs.ai distinguishes itself as a leading provider. Their innovative solutions address the key challenges faced by businesses looking to leverage AI technologies effectively.
Why Choose Keylabs.ai?
Keylabs.ai offers a comprehensive suite of features designed to facilitate the entire data annotation process:
- AI-Powered Automation: The platform employs advanced AI algorithms to assist in the annotation process, reducing time spent on manual labeling.
- Customizable Workflows: Users can tailor the annotation workflows to fit specific project requirements, enhancing efficiency.
- High-Quality Data: With an emphasis on maintaining data integrity, Keylabs.ai ensures that every annotation meets strict quality standards.
- Scalable Solutions: Whether you’re a startup or a large enterprise, Keylabs.ai can scale according to your data annotation needs.
- Dedicated Support: Their expert team is available to assist clients throughout the data annotation process, ensuring a seamless experience.
The Process of Choosing a Data Annotation Platform
Selecting the right data annotation platform is pivotal for any organization embarking on an AI project. Here are the essential steps to guide you through the selection process:
1. Assess Your Requirements
Identify the types of data you need to annotate and the scale of your project. Understanding your specific needs is the first step in choosing the right tool.
2. Research Available Tools
Conduct thorough research on various data annotation tools and platforms available in the market, focusing on their features and benefits.
3. Evaluate User Feedback
Look for reviews and testimonials from other users. Real-world experiences can provide valuable insights into the tool’s performance and reliability.
4. Request Demos
Most reputable data annotation platforms, like Keylabs.ai, offer free demos or trials. Take advantage of these opportunities to evaluate the tool firsthand.
5. Consider Pricing Models
Evaluate the pricing structures of different platforms. Compare the features offered at each price point to determine the best value for your budget.
Best Practices for Data Annotation
- Define Clear Guidelines: Providing annotators with clear instructions ensures consistency and quality across the dataset.
- Use Sample Data: Conducting pilot tests with sample data can help identify potential issues before scaling up the annotation process.
- Incorporate Feedback: Regularly solicit feedback from annotators and stakeholders to refine processes and guidelines.
- Monitor Progress: Use dashboards or tools to track the progress of your annotation tasks and ensure deadlines are met.
- Continuously Improve: Analyze the outcomes of previous projects and apply lessons learned to improve future annotation efforts.
Future Trends in Data Annotation
The landscape of data annotation is ever-evolving, influenced by advancements in technology and the growing demands of the AI industry. Here are some expected trends that will shape the future of data annotation:
1. Increased Automation
As AI technologies advance, we can expect tools to become even more automated, streamlining the data annotation process further and reducing human intervention.
2. Collaborative Annotation Platforms
There will be a rise in platforms that support collaborative annotation efforts, allowing teams from different locations to work together in real-time.
3. Enhanced AI Features
Data annotation tools will incorporate more sophisticated AI features, enabling them to learn from user inputs and improve their annotation capabilities over time.
4. Focus on Data Privacy
With increased scrutiny around data privacy, annotation platforms will need to implement stricter data protection protocols to ensure compliance with regulations.
Conclusion
The world of data annotation tools and data annotation platforms is critical in the development of AI and machine learning technologies. As organizations seek to leverage AI's potential, the importance of high-quality annotated data will only continue to grow. Platforms like Keylabs.ai provide scalable, efficient, and comprehensive solutions to meet these needs. By selecting the right tools and implementing best practices, businesses can ensure they stay ahead in the rapidly evolving technological landscape, unlocking the full potential of their data for future innovations.
https://keylabs.ai/