Labeling Tools for Machine Learning: A Comprehensive Guide
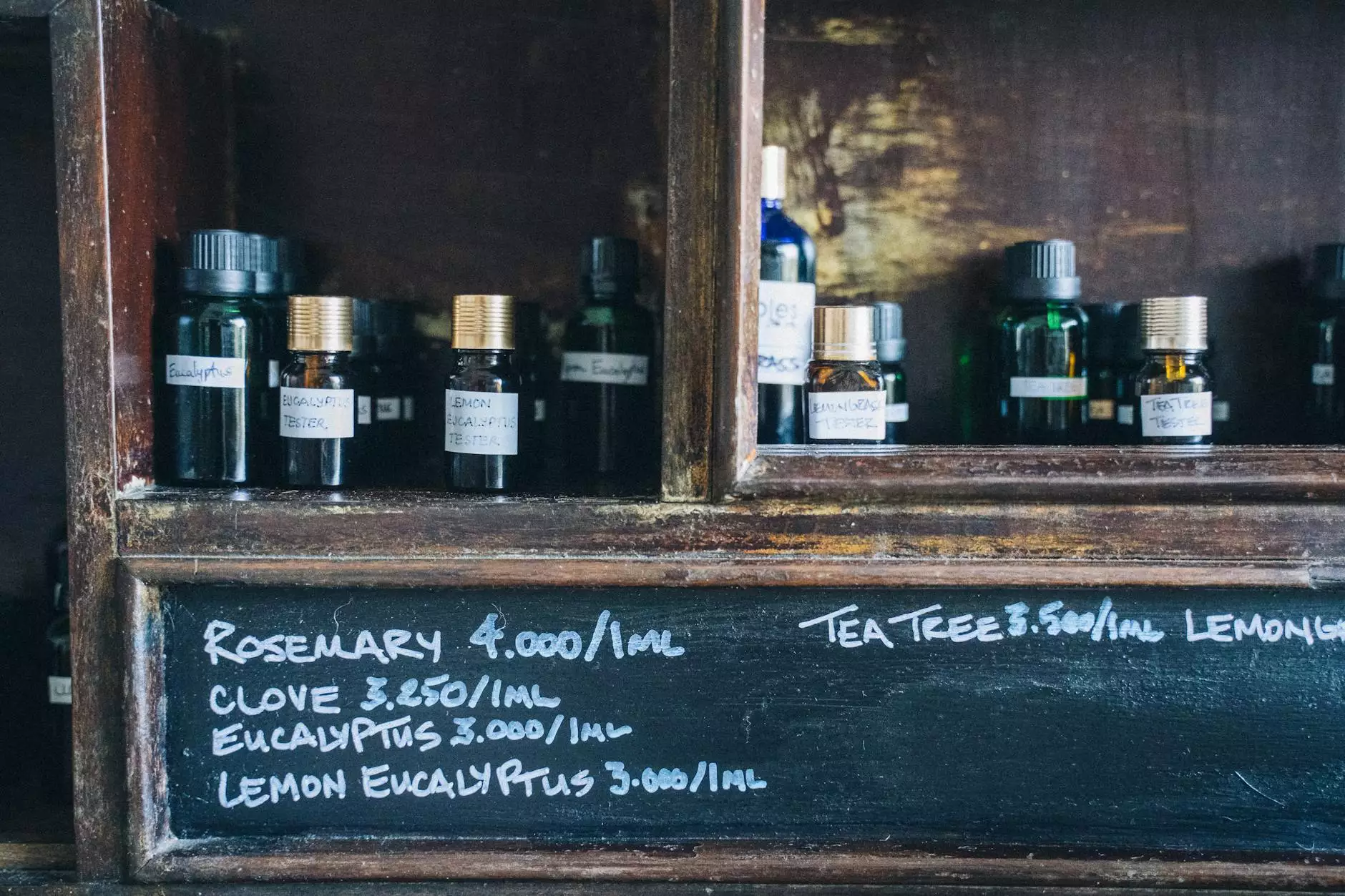
In the age of artificial intelligence, labeling tools for machine learning have become essential for businesses that aim to leverage data effectively. As AI applications grow, the demand for accurate data annotation continues to rise. In this article, we will delve into the intricate world of data annotation tools, examining their significance, functionalities, and the unique offerings available at Keylabs.ai.
Understanding the Importance of Data Annotation
Data annotation is the process of labeling data—be it text, images, or videos—to enable machine learning models to learn effectively. This ensures that AI systems can make accurate predictions and decisions based on the data they process. The quality and accuracy of these annotations are critical as they influence the performance of machine learning models.
What are Labeling Tools for Machine Learning?
Labeling tools are software solutions designed to facilitate the annotation of datasets. These tools can vary in complexity and functionality but at their core, they aim to streamline the data labeling process, making it easier and faster for organizations to prepare datasets for training AI models.
Key Features of Effective Labeling Tools
When considering labeling tools for machine learning, several key features distinguish the best from the rest:
- User-Friendly Interface: An intuitive interface that allows users to annotate data easily and efficiently without extensive training.
- Multiple Data Formats: Support for various data types, including images, text, audio, and video, catering to diverse project requirements.
- Collaboration Features: Options for team collaboration where multiple users can annotate data in real-time while maintaining version control.
- Quality Assurance Mechanisms: Tools that provide validation and quality checks to ensure annotation accuracy.
- Integration Capabilities: Seamless integration with machine learning frameworks to facilitate a smooth workflow from data collection to model training.
- Automation Options: Use of AI to assist with or automate parts of the labeling process, reducing labor time and errors.
The Role of Keylabs.ai in Data Annotation
Keylabs.ai offers a robust data annotation platform that stands out in the realm of labeling tools for machine learning. By integrating cutting-edge technology with a user-centric design, Keylabs.ai enhances the efficiency and accuracy of data annotation processes.
Why Choose Keylabs.ai?
Here are some compelling reasons why businesses should consider Keylabs.ai as their go-to data annotation tool:
- High Efficiency: The platform is designed to maximize productivity, allowing teams to annotate thousands of data points accurately within a minimal timeframe.
- Scalable Solutions: Suitable for projects of any size, Keylabs.ai accommodates the needs of both small startups and large enterprises.
- Customizable Workflows: Users can tailor workflows according to specific project requirements, ensuring that every labeling task meets the unique needs of their AI applications.
- Real-Time Collaboration: With collaborative features, teams can work together seamlessly, making it easier to manage large annotation projects.
- Advanced AI Assistance: Keylabs.ai utilizes AI technology to assist with data labeling, significantly reducing the time and effort required for manual annotation.
Types of Labeling Tools Available
Labeling tools for machine learning can be broadly categorized based on the type of data they handle. Here are some common types:
Image Annotation Tools
Image annotation is crucial for computer vision models. Features often include:
- Bounding Box Annotations: Used to highlight and classify objects within an image.
- Semantic Segmentation: Pixel-level labeling that classifies each pixel into categories for detailed image understanding.
- Image Classification: Labels entire images based on the dominant object or action.
Text Annotation Tools
Text annotation plays a vital role in natural language processing (NLP). Key features include:
- Entity Recognition: Identifying and classifying named entities (e.g., names, dates) within the text.
- Sentiment Annotation: Assigning sentiment labels (positive, negative, neutral) to text segments.
- Text Categorization: Classifying text into predefined categories for efficient data management.
Audio Annotation Tools
For applications involving speech recognition, audio annotation tools provide features such as:
- Transcription: Converting spoken language into written text and labeling specific terms or phrases.
- Speaker Identification: Labeling speakers in audio files for multi-speaker scenarios.
- Emotion Tagging: Annotating audio segments based on the emotion conveyed by the speaker.
Video Annotation Tools
Video annotation tools allow for comprehensive labeling of video content. Key functionalities include:
- Object Tracking: Monitoring the movement of objects across frames for applications such as autonomous vehicles.
- Action Recognition: Labeling actions taken by individuals within a video.
- Scene Detection: Identifying and categorizing different scenes throughout the video.
Challenges in Data Annotation and How to Overcome Them
Despite the technological advancements in labeling tools for machine learning, challenges remain in the data annotation process:
Maintaining Annotation Quality
Ensuring high-quality annotations can be challenging, especially with large datasets. Businesses can adopt the following strategies:
- Implement quality control measures such as regular audits of annotated data.
- Use multiple annotators for cross-validation, ensuring that different perspectives contribute to the final label.
- Invest in ongoing training and support for annotators to improve their skills and understanding of project requirements.
Scarcity of Skilled Annotators
Finding skilled annotators can be a significant hurdle. Companies can address this through:
- Leveraging AI assistance to reduce the workload on human annotators.
- Utilizing platforms like Keylabs.ai that can source annotators from a larger talent pool.
- Creating a structured training program to cultivate in-house talent.
Future Trends in Labeling Tools for Machine Learning
The field of labeling tools for machine learning is rapidly evolving. Here are some trends we can expect to see:
Increased Automation
As AI technologies continue to improve, automation in the labeling process will become more prevalent. Tools will incorporate machine learning algorithms that can predict labels based on minimal user input, drastically reducing the time required for data preparation.
Crowdsourced Annotations
Crowdsourcing is likely to gain traction as a method for obtaining large datasets with diverse inputs. Platforms that connect businesses to skilled annotators globally will become more common, offering scalability and flexibility.
Enhanced Collaboration Features
Future labeling tools will increasingly focus on enhancing collaboration, making it easier for teams to work together in real-time. Features such as integrated messaging, video tutorials, and automated feedback mechanisms will facilitate better communication among team members.
Conclusion
In summary, labeling tools for machine learning are crucial for the successful deployment of AI technologies. Businesses looking to enhance their data annotation processes will find that platforms like Keylabs.ai not only meet but exceed their expectations. With user-friendly interfaces, advanced automation, and comprehensive support for various data formats, Keylabs.ai provides an unparalleled solution for modern data challenges. By investing in the right labeling tools, companies can significantly upgrade their machine learning capabilities, paving the way for innovative applications in the future.